Business intelligence in insurance industry is revolutionizing how insurers operate, from pricing strategies to risk management. This detailed analysis explores how data-driven insights are transforming traditional insurance operations, enhancing customer service, and optimizing claims processing.
The increasing availability of data, coupled with advancements in technology, has created unprecedented opportunities for insurers to leverage business intelligence. This overview will explore the key components of successful BI implementation in the insurance sector, including data sources, tools, and the challenges involved.
Introduction to Business Intelligence in Insurance
Business intelligence (BI) in the insurance industry encompasses the use of data analysis and reporting tools to transform raw data into actionable insights. This process facilitates better understanding of customer behavior, market trends, and operational efficiency, leading to improved decision-making and strategic planning. BI leverages various technologies and analytical techniques to extract value from vast datasets, ultimately enhancing profitability and risk management within insurance organizations.Traditional insurance operations often rely on historical data and expert judgment.
BI-driven approaches, on the other hand, go beyond these limitations by utilizing sophisticated algorithms and predictive modeling. This allows for a more comprehensive understanding of complex relationships within the data, enabling the identification of patterns and anomalies that traditional methods might miss. The result is a more data-driven, informed, and agile approach to underwriting, claims processing, and customer service.Data-driven insights significantly enhance decision-making in insurance.
By analyzing customer demographics, claims history, and policy behaviors, insurance companies can identify high-risk individuals or groups, adjust pricing strategies accordingly, and develop targeted marketing campaigns. Furthermore, predictive analytics can anticipate future claims costs, allowing for proactive risk management and investment strategies. For instance, a life insurance company could use BI to identify trends in customer health, enabling them to offer personalized preventative care packages.Technology plays a crucial role in enabling BI implementation in insurance.
Advanced analytics tools, data warehousing systems, and cloud-based platforms are essential for managing and analyzing the vast amounts of data generated by insurance companies. Data visualization tools, such as dashboards and interactive reports, make it easier to understand and communicate insights to stakeholders. The increasing availability of powerful computing resources and sophisticated algorithms has dramatically improved the ability of insurance companies to extract actionable intelligence from their data.
Different Types of Insurance and BI Application
Applying business intelligence across various insurance sectors offers distinct advantages. The diverse nature of policies requires tailored approaches to leverage BI effectively.
Insurance Type | BI Application Examples |
---|---|
Life Insurance | Predicting mortality rates based on health data, assessing risk factors, and optimizing pricing models. Analyzing customer behavior and preferences to personalize offerings. |
Health Insurance | Identifying trends in healthcare costs, predicting future claims, and tailoring preventative care programs. Assessing the effectiveness of different treatment options and analyzing customer satisfaction. |
Property Insurance | Analyzing historical weather patterns and building data to assess risk for specific locations. Predicting potential claims based on geographic factors and building codes. Analyzing claims data to identify fraudulent activities and optimize claim settlement processes. |
Data Sources and Collection in Insurance BI
Business intelligence (BI) in the insurance industry relies heavily on the effective collection, integration, and analysis of diverse data sources. This crucial process allows insurers to understand customer behavior, predict risks, optimize pricing strategies, and enhance operational efficiency. Accurate and reliable data is paramount to achieving meaningful insights and driving informed decision-making.Data sources are varied and encompass a wide range of information, including claims data, customer demographics, market trends, and external factors like economic indicators.
Analyzing trends in the insurance industry using business intelligence is crucial for profitability. One particularly intriguing case study, situated at a rather picturesque address like 2 62 salmon st port melbourne , demonstrates the potential for leveraging data to optimize risk assessment and policy pricing. Ultimately, this highlights the indispensable role of business intelligence in navigating the complexities of the insurance market.
Proper management and analysis of these sources are essential for building robust BI systems that support the strategic objectives of insurance companies.
Data Sources in Insurance BI, Business intelligence in insurance industry
Insurance BI systems leverage a multitude of data sources to gain comprehensive insights. These sources include claims data, customer data, market data, and internal operational data. Claims data provides insights into the frequency and severity of events, while customer data offers a detailed profile of policyholders. Market data includes information about industry trends, competitor activities, and economic conditions.
Internal operational data encompasses various aspects of the insurance company’s internal processes, such as sales, underwriting, and customer service.
Data Quality and its Impact on BI Insights
Data quality is paramount in insurance BI. Inaccurate, incomplete, or inconsistent data can lead to flawed analyses and incorrect conclusions. This can result in poor decision-making, potentially impacting profitability and customer satisfaction. Ensuring data quality involves rigorous validation, standardization, and cleaning procedures. For instance, inconsistencies in policyholder addresses or incorrect claim amounts can significantly distort analysis results, leading to inaccurate risk assessments and inappropriate pricing strategies.
Robust data quality management systems are critical for generating reliable and actionable insights.
Data Integration and Transformation
Data integration is a crucial step in building a BI system. This process combines data from various sources into a unified format. Data transformation converts data into a suitable format for analysis. Data transformation steps can include data cleaning, standardization, and aggregation. These processes are essential for ensuring that data from diverse sources can be combined and analyzed efficiently.
For example, merging claims data with customer demographic data allows for the identification of patterns and trends in claim frequency and severity associated with specific customer segments. This integration and analysis can lead to tailored risk assessment and pricing strategies.
Data Collection Methods in Different Insurance Sectors
The methods of data collection vary depending on the specific insurance sector. Life insurance companies may collect data on mortality rates, while property and casualty insurers gather data on claims related to accidents and damages. Health insurance companies collect data on medical expenses and treatment patterns. The methods for data collection can range from structured databases to surveys, and often utilize sophisticated algorithms for processing and interpreting the information.
Furthermore, data collection strategies should adhere to privacy regulations and ethical considerations to protect sensitive information.
Data Security Measures for Insurance Data
- Data Encryption: Encrypting sensitive data, such as customer financial information and claim details, protects it from unauthorized access. This is crucial for maintaining data confidentiality and compliance with regulations like GDPR.
- Access Control: Implementing robust access controls limits access to sensitive data to authorized personnel only. This prevents unauthorized individuals from accessing and modifying sensitive information, which is a key element of data security.
- Data Backup and Recovery: Regular data backups and recovery procedures safeguard against data loss due to hardware failures, cyberattacks, or human errors. Having a well-defined disaster recovery plan ensures business continuity.
- Regular Security Audits: Regular security audits and vulnerability assessments help identify and address potential security weaknesses in the data infrastructure. This proactive approach is vital for maintaining a robust security posture.
Data Type | Encryption Method | Access Control | Backup Frequency | Audit Frequency |
---|---|---|---|---|
Customer Financial Data | Advanced Encryption Standard (AES) | Multi-factor authentication | Daily | Quarterly |
Claims Data | AES-256 | Role-based access control | Weekly | Semi-annually |
Policyholder Information | Symmetric Encryption | Password protection | Monthly | Annually |
Data security measures are essential to protect sensitive information and maintain customer trust. A robust data security framework is essential for insurance companies to protect sensitive data from unauthorized access and ensure compliance with industry regulations.
BI Tools and Technologies for Insurance: Business Intelligence In Insurance Industry
Business intelligence (BI) tools are critical for insurance companies to gain insights from their data, enabling strategic decision-making, improved risk assessment, and enhanced customer service. These tools facilitate the analysis of vast datasets, transforming raw information into actionable intelligence that drives profitability and competitiveness. The selection of appropriate BI tools is crucial, as different tools cater to various analytical needs and technological infrastructure.Effective implementation of BI tools in the insurance sector necessitates a thorough understanding of the specific requirements of the insurance business.
These tools empower insurers to analyze complex data, identifying patterns and trends that might otherwise remain hidden. This understanding is crucial for proactive risk management, customer segmentation, and product development, all contributing to a competitive advantage in the insurance market.
Key Software Tools and Technologies
Various software tools and technologies are employed in insurance BI, each serving specific analytical purposes. These tools range from traditional reporting and data warehousing solutions to advanced predictive analytics and machine learning platforms. The choice of tools often depends on the company’s existing infrastructure, data volume, and analytical needs. Companies frequently leverage cloud-based platforms for scalability and cost-effectiveness.
Examples of Analytical Platforms
Several analytical platforms are commonly used in the insurance sector. These platforms provide a comprehensive suite of tools for data analysis, reporting, and visualization. Examples include Qlik Sense, Tableau, Power BI, and various specialized insurance BI solutions offered by vendors such as SAS and IBM. These platforms facilitate the creation of interactive dashboards, enabling real-time monitoring of key performance indicators (KPIs) and the identification of emerging trends.
Role of Cloud Computing
Cloud computing plays a significant role in supporting insurance BI initiatives. Cloud-based solutions offer scalability, flexibility, and cost-effectiveness, allowing insurance companies to process vast datasets and deploy advanced analytics without significant upfront investment in hardware and infrastructure. Cloud platforms enable faster deployment of BI solutions and greater accessibility for data analysis across various departments within the organization. The cloud environment facilitates collaborative data analysis and fosters data sharing among different teams.
Comparison of BI Tools
Different BI tools cater to varying needs and analytical capabilities. Some tools excel at reporting and data visualization, while others are more adept at complex predictive modeling. Factors to consider when comparing tools include the tool’s capabilities for handling large datasets, its integration with existing systems, its user-friendliness, and its scalability to accommodate future growth. Open-source tools often offer cost-effectiveness but may require greater technical expertise for implementation and maintenance.
Table of Popular BI Tools
Tool | Features | Functionalities |
---|---|---|
Qlik Sense | Interactive dashboards, data visualization, advanced analytics | Data exploration, reporting, forecasting, ad-hoc analysis |
Tableau | Visual data exploration, interactive dashboards, data blending | Data visualization, reporting, data storytelling, predictive modeling |
Power BI | Intuitive interface, integration with Microsoft ecosystem, data visualization | Data visualization, reporting, dashboards, data analysis, integration with other Microsoft products |
SAS | Advanced statistical modeling, predictive analytics, data mining | Statistical modeling, predictive analytics, data mining, risk management |
Business Applications of BI in Insurance
Business intelligence (BI) is transforming the insurance industry by providing a comprehensive view of data, enabling informed decision-making across various operational areas. This data-driven approach empowers insurers to optimize processes, enhance customer experiences, and mitigate risks, ultimately leading to improved profitability and market competitiveness. Leveraging BI tools and techniques allows insurers to extract actionable insights from vast datasets, leading to strategic adjustments in pricing, risk management, customer service, claims processing, and fraud detection.
Pricing Strategies Enhancement
BI facilitates data-driven pricing strategies by analyzing historical claim data, demographics, risk factors, and market trends. Insurers can identify patterns and correlations to refine pricing models and ensure premiums accurately reflect the true risk associated with individual policies. This approach leads to more competitive pricing, improved profitability, and a better customer experience by aligning premiums with actual risk profiles.
For example, an insurer might use BI to determine that drivers in a specific geographic area have a higher accident rate, justifying higher premiums for those drivers while offering discounted rates to drivers with lower accident histories.
Risk Assessment and Management
BI empowers insurers to proactively assess and manage risks through the identification of key risk indicators (KRIs). By analyzing large datasets encompassing various factors such as demographics, driving behavior, and claims history, insurers can build predictive models that forecast future risk levels. This allows for proactive risk mitigation strategies and informed decision-making regarding policy underwriting, investment diversification, and overall portfolio management.
For example, an insurer can identify trends in claims data related to specific vehicle types or geographical locations, enabling targeted risk mitigation efforts and investment strategies.
Customer Service and Retention
BI improves customer service and retention by providing a holistic view of customer interactions and preferences. Analyzing customer interactions, claims history, and feedback allows insurers to tailor services to individual needs and preferences, leading to increased customer satisfaction and loyalty. Personalized communication strategies, tailored service offerings, and proactive support are crucial for enhancing customer relationships. For instance, BI can identify customers who are at risk of canceling their policies and offer targeted incentives or personalized service packages to retain them.
Claims Processing Optimization
BI streamlines claims processing by automating tasks, identifying bottlenecks, and optimizing workflows. Insurers can use BI to analyze claims data to identify patterns and inefficiencies in the claims handling process. This allows for the development of optimized workflows, improved processing times, and enhanced customer satisfaction. BI tools can automatically process routine claims, reducing manual intervention and accelerating the claims settlement process.
For example, an insurer might use BI to identify a significant delay in processing claims related to specific types of damage, enabling them to implement process improvements and reduce turnaround time.
Fraud Detection and Prevention
BI facilitates fraud detection and prevention by identifying suspicious patterns and anomalies in claims data. Sophisticated BI algorithms can analyze vast amounts of data, including policy details, claim information, and customer behavior, to detect fraudulent activities. Implementing advanced analytics techniques allows insurers to proactively identify and prevent fraudulent claims, protecting the company’s financial interests and maintaining customer trust.
For example, an insurer might use BI to identify unusual claim patterns that deviate significantly from historical trends, raising flags for potential fraudulent activity and triggering further investigation.
Challenges and Considerations in Implementing BI
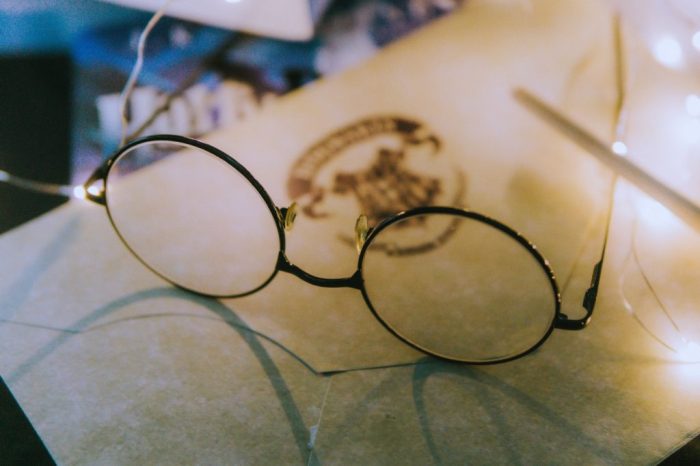
Implementing Business Intelligence (BI) systems in the insurance industry presents unique challenges, requiring careful consideration of data management, technical capabilities, and organizational factors. These challenges are often intertwined, requiring a holistic approach to overcome them effectively. Successful BI implementation hinges on a thorough understanding of these obstacles and a proactive strategy for addressing them.
Common Challenges in Implementing BI
Insurance companies face numerous hurdles in implementing BI solutions. These range from the complexity of insurance data to the need for significant organizational change. Data volume, variety, and velocity are often overwhelming, necessitating robust data warehousing and processing capabilities. Integrating disparate data sources, ensuring data quality, and establishing a consistent data model can be complex and time-consuming.
- Data Integration Challenges: Insurance data is often scattered across various systems and formats, making integration a significant hurdle. Legacy systems, proprietary formats, and inconsistent data structures pose integration challenges. Companies must develop robust ETL (Extract, Transform, Load) processes to consolidate and harmonize data from diverse sources.
- Data Quality Issues: Inaccurate, incomplete, or inconsistent data can lead to flawed analyses and unreliable insights. Ensuring data quality throughout the BI lifecycle is crucial. Companies must implement data validation rules, cleansing procedures, and monitoring mechanisms to maintain data integrity.
- Resistance to Change: Implementing BI often requires significant organizational change. Employees may be resistant to adopting new processes or relinquishing traditional methods. Companies need to address this resistance through clear communication, training programs, and demonstrating the value of BI.
- Cost Considerations: BI implementations can be expensive, involving significant upfront investments in software, hardware, and personnel. The costs of data warehousing, data modeling, and ongoing maintenance should be factored into the overall budget.
Data Governance and Compliance
Data governance and compliance are paramount in the insurance industry. Maintaining the integrity and security of sensitive customer data is crucial, requiring strict adherence to regulatory requirements. Compliance with regulations like GDPR, HIPAA, and others must be factored into BI system design and operation. A robust data governance framework is essential to ensure data quality, security, and regulatory compliance.
- Regulatory Compliance: Insurance companies must comply with stringent regulatory requirements related to data privacy and security. BI systems must be designed and operated in accordance with these regulations. Failure to comply can result in significant penalties and reputational damage.
- Data Security: Protecting sensitive customer data is critical. BI systems must implement robust security measures to prevent unauthorized access and data breaches. Data encryption, access controls, and regular security audits are essential components of a comprehensive security strategy.
- Data Ownership and Responsibility: Clearly defining data ownership and responsibility within the organization is essential. This ensures accountability for data quality, security, and compliance. A well-defined data governance framework establishes clear roles and responsibilities for managing data.
Skilled Personnel
Implementing and maintaining BI systems requires a dedicated team with specialized skills. Data analysts, data scientists, and BI developers are essential to extract insights, build models, and ensure the system’s functionality. Training and development are crucial for personnel to adapt to new technologies and approaches.
- Data Analysis Expertise: Insurance BI projects require professionals proficient in data analysis techniques, including statistical modeling, data visualization, and predictive analytics. This ensures accurate interpretation of data and reliable insights.
- Technical Expertise: Developing, implementing, and maintaining BI systems requires individuals with expertise in database management, data warehousing, and ETL processes. Technical personnel are critical to the system’s architecture and functionality.
- BI Project Management: Managing BI projects effectively requires professionals with experience in project planning, execution, and monitoring. This includes understanding project timelines, budgets, and resource allocation.
Cost Considerations
The cost of implementing BI solutions encompasses various elements, from initial setup to ongoing maintenance. The total cost of ownership (TCO) should be considered, including software licenses, hardware costs, personnel salaries, training, and potential penalties for non-compliance. Companies should conduct thorough cost-benefit analyses before implementing a BI solution.
- Software and Hardware Costs: Licensing fees for BI software and hardware infrastructure are significant upfront costs. Companies must evaluate the costs of different solutions based on their specific needs and scale.
- Personnel Costs: Hiring and training skilled personnel for data analysis, development, and project management is an ongoing expense. Compensation and benefits for these roles should be factored into the budget.
- Data Management Costs: Data warehousing, data integration, and data governance require dedicated resources and ongoing costs. These should be estimated and factored into the TCO.
Ongoing Maintenance and Updates
BI systems require continuous maintenance and updates to ensure their effectiveness and relevance. Regular system upgrades, data refreshes, and security patches are necessary. This includes maintaining data quality, addressing emerging challenges, and staying abreast of technological advancements. Failure to maintain systems can lead to decreased efficiency and outdated insights.
- System Upgrades and Maintenance: BI systems require regular updates to ensure compatibility with evolving technologies and data sources. Maintaining system performance and stability through regular maintenance is essential.
- Data Refresh and Updates: BI systems depend on fresh data for reliable insights. Establishing regular data refresh schedules and procedures is critical to maintain the system’s accuracy.
- Security Patching: BI systems need to be protected against evolving security threats. Regular security patching is crucial to ensure data integrity and confidentiality.
Case Studies and Examples of Insurance BI Success
Business intelligence (BI) implementations in the insurance industry are increasingly demonstrating tangible benefits, driving operational efficiency and boosting profitability across various segments. These successful deployments showcase the power of data-driven decision-making in optimizing processes, identifying emerging trends, and mitigating risks. The following case studies highlight specific examples of successful BI implementations.Successful BI implementations in insurance companies often leverage data from diverse sources to provide a holistic view of the business.
This integrated view allows for improved risk assessment, personalized customer service, and proactive fraud detection. The subsequent sections provide detailed examples of these implementations, showcasing their impact.
Analyzing trends in the insurance sector using business intelligence is crucial for profitability. However, sometimes the complexities of actuarial calculations can feel akin to mastering the subtle nuances of power shots in Mario Tennis: Power Tour ROM mario tennis: power tour rom. Ultimately, harnessing data-driven insights remains paramount for success in the insurance industry.
Successful BI Implementations in Property and Casualty Insurance
Insurance companies often face significant challenges in managing claims, assessing risk, and pricing policies accurately. Business intelligence solutions can provide invaluable insights to address these challenges. For instance, a large property and casualty insurer implemented a BI platform to analyze historical claim data. This analysis revealed patterns in claim frequency and severity, enabling the company to identify high-risk policyholders and adjust premiums accordingly.
The result was a significant reduction in claim payouts and an improvement in profitability. Another example involves a company that used BI to identify geographic clusters with higher-than-average claim rates. This enabled them to deploy targeted risk mitigation strategies in those areas, leading to improved efficiency in claims handling.
Successful BI Implementations in Life Insurance
In the life insurance sector, BI plays a crucial role in understanding customer behavior and optimizing product offerings. A life insurer used BI to analyze customer data and identify factors associated with policy lapses. By understanding the drivers behind these lapses, the company was able to implement targeted retention strategies, resulting in a noticeable increase in policy retention rates.
Further, a life insurer used BI to evaluate the effectiveness of various marketing campaigns. This analysis helped the company to optimize its marketing strategies, ultimately increasing sales and profitability.
Comparative Case Studies of Insurance BI Success
Insurance Provider | Insurance Segment | BI Implementation Focus | Key Outcomes |
---|---|---|---|
Acme Insurance | Property & Casualty | Claims processing optimization, risk assessment | Reduced claim processing time by 20%, decreased claim payouts by 15%, and improved underwriting accuracy |
Global Life Insurance | Life Insurance | Customer retention, product optimization | Increased policy retention rate by 12%, improved customer segmentation, and enhanced product profitability |
Prosperity Insurance | Health Insurance | Fraud detection, premium optimization | Reduced fraudulent claims by 10%, improved premium pricing accuracy, and enhanced customer service response time |
The table above presents comparative case studies, highlighting successful BI implementations across various insurance segments. These implementations showcase how BI can be leveraged to achieve significant improvements in operational efficiency and profitability. Different insurance providers have tailored their BI solutions to address specific business needs within their respective segments.
Future Trends and Developments in Insurance BI
The insurance industry is undergoing a period of rapid transformation, driven by technological advancements and evolving customer expectations. Business Intelligence (BI) plays a crucial role in navigating this change, enabling insurers to leverage data effectively to enhance operational efficiency, improve decision-making, and gain a competitive edge. This evolution necessitates a proactive approach to understanding and adapting to future trends shaping the landscape of insurance BI.
Emerging Technologies Influencing Insurance BI
Advancements in technology are reshaping the way insurers collect, analyze, and utilize data. Cloud computing, big data analytics, and artificial intelligence are key drivers of this transformation, empowering insurers to derive actionable insights from massive datasets and enhance their overall operational efficiency. The integration of these technologies with existing BI systems is critical for future success.
Artificial Intelligence and Machine Learning in Insurance BI
Artificial intelligence (AI) and machine learning (ML) are revolutionizing insurance BI. AI-powered systems can analyze vast amounts of data to identify patterns, predict future outcomes, and automate tasks. Examples include: automated claims processing, fraud detection, risk assessment, and personalized pricing models. By automating repetitive tasks, AI allows insurance professionals to focus on more strategic initiatives.
- Automated Claims Processing: AI algorithms can analyze claim data to identify fraudulent activities or inconsistencies, streamlining the claims process. This reduces processing time and ensures accurate payouts while detecting and mitigating potential fraud.
- Fraud Detection: Machine learning models can analyze transactional data to identify suspicious patterns, flag potentially fraudulent claims, and reduce the overall cost of insurance fraud.
- Risk Assessment: AI algorithms can evaluate a multitude of risk factors to assess an individual’s risk profile more accurately than traditional methods, enabling personalized insurance pricing.
- Personalized Pricing Models: AI can use customer data to create highly customized pricing models that account for various factors, allowing insurers to better tailor their offerings to individual customer needs. This improves customer satisfaction and potentially increases revenue.
Impact of Increasing Data Availability on Insurance BI
The exponential growth of data, encompassing structured and unstructured information, presents both opportunities and challenges for insurance BI. Insurers must develop robust strategies for data collection, storage, and analysis to effectively leverage the increasing availability of data. This encompasses a wide range of data sources, including social media, IoT devices, and customer interactions.
Big Data Analytics Transforming Insurance Operations
Big data analytics provides insurers with the tools to uncover hidden patterns and trends within vast datasets, enabling them to make more informed decisions. By leveraging big data analytics, insurers can:
- Improve risk assessment: Analyze extensive data sets to refine risk profiles and establish more accurate pricing models. This can lead to more competitive premiums for lower-risk customers and enhanced profitability.
- Enhance customer segmentation: Identify different customer segments and tailor products and services accordingly. This fosters customer loyalty and engagement. A clear understanding of diverse customer needs is vital.
- Optimize operational efficiency: Analyze operational data to identify bottlenecks and inefficiencies, leading to better resource allocation and cost reduction.
Role of Blockchain in Future BI Implementations
Blockchain technology, known for its security and transparency, holds significant potential for enhancing insurance BI. Blockchain can improve data integrity, traceability, and security, facilitating secure and reliable data sharing among different stakeholders.
- Improved Data Integrity: Blockchain’s decentralized nature ensures data immutability, preventing unauthorized modifications and enhancing trust among stakeholders. This can strengthen the integrity of claims data and other critical records.
- Enhanced Traceability: Blockchain facilitates the tracking of insurance policies and claims throughout their lifecycle, improving transparency and accountability. This is vital in processes like claim settlement and policy issuance.
- Improved Security: The decentralized nature of blockchain makes it significantly more resistant to cyberattacks, safeguarding sensitive data. This protection is crucial for insurers dealing with substantial amounts of sensitive customer information.
Summary
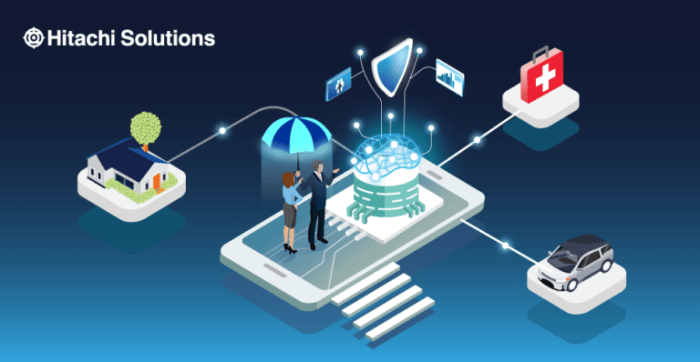
In conclusion, business intelligence is no longer a futuristic concept but a critical component of success in the modern insurance industry. By embracing data-driven strategies, insurers can enhance their decision-making processes, improve operational efficiency, and foster stronger customer relationships. The future of insurance is inextricably linked to effective business intelligence implementation.
Question Bank
What are some common data sources used in insurance BI?
Common data sources include claims data, customer data, market data, and industry benchmarks. Data quality is crucial for accurate insights.
What are the primary challenges in implementing BI in insurance?
Challenges include data governance and compliance, the need for skilled personnel, and cost considerations related to implementing and maintaining BI systems.
How does AI/ML contribute to insurance BI?
AI and machine learning are increasingly used for tasks like fraud detection, risk assessment, and personalized pricing models. This automation and advanced analysis are critical in a data-heavy industry like insurance.
How does data security relate to BI implementation?
Data security is paramount. Insurance companies must implement robust security measures to protect sensitive customer and financial data, ensuring compliance with regulations like GDPR.